The bigger the swirl, the bigger the problem — and the bigger the computing power needed to solve it.
Computational fluid dynamics researchers have spent decades studying how liquids and gases flow in and around such machinery as airplane wings, propeller blades and jet engines in search of faster speeds and more efficient aerodynamics. Existing mathematical models tend to work fine when studying the calmer parts of those flows, known as the laminar regions.
But those models too often break down when applied to the swirling eddies and currents, known as turbulence, produced by those fluids at the crucial points of contact, such as a wing’s surface or an engine’s inner walls.
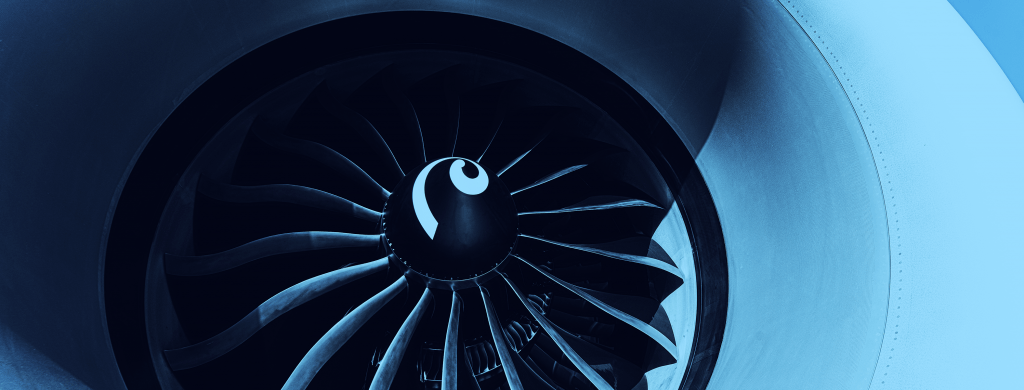
Simulations of turbulence performed on Oak Ridge National Laboratory’s Summit supercomputer by GE and ORNL researchers could lead to better aircraft designs, environmentally cleaner flights and savings of as much as $400 million per year.
Credit: Getty Images
GE, maker of some of the world’s most powerful jet engines and gas-powered turbines, sought to better understand and predict the impact of that turbulence through more advanced modeling and simulation. Turbulence routinely impedes jet engine efficiency, leading to hundreds of millions of dollars burned per year in wasted jet fuel alone.
Studies with experts from the U.S. Department of Energy’s Oak Ridge National Laboratory and simulations run on one of the world’s most powerful supercomputers led GE researchers to a solution that could save airlines more than 125 million gallons of jet fuel annually and reduce yearly greenhouse gas emissions by 850,000 metric tons — the equivalent of $400 million. Finding that solution took breaking down longstanding barriers to better understanding old questions about turbulence and its impact.
“It’s called the ‘near wall’ problem,” said Stephan Priebe, a computational scientist for GE Research. “The flow around these objects creates turbulence with cascading eddies that are challenging to predict and model. Look at a cloud, for example, and you’ll see a big structure. Look closer, and you’ll see smaller structures within that structure, and if you look even closer, you’ll see even smaller structures within those, and so on. That’s what we’re dealing with here.”
Priebe and his team sought to capture that turbulence in a simulation detailed enough to predict likely flows and to use those findings to improve the models employed in GE’s jet engine design. Existing approaches weren’t up to the challenge.
“In realistic conditions, such as inside a jet engine, the actual range of scales of the turbulent eddies is very wide,” Priebe explained. “We can try to model these eddies computationally, but the amount of resolution we need becomes very time-consuming and computationally demanding very quickly — to the point it’s beyond our computing capacity or the capacity of most computers. We can’t adjust our designs to solve for a problem we can’t predict.”
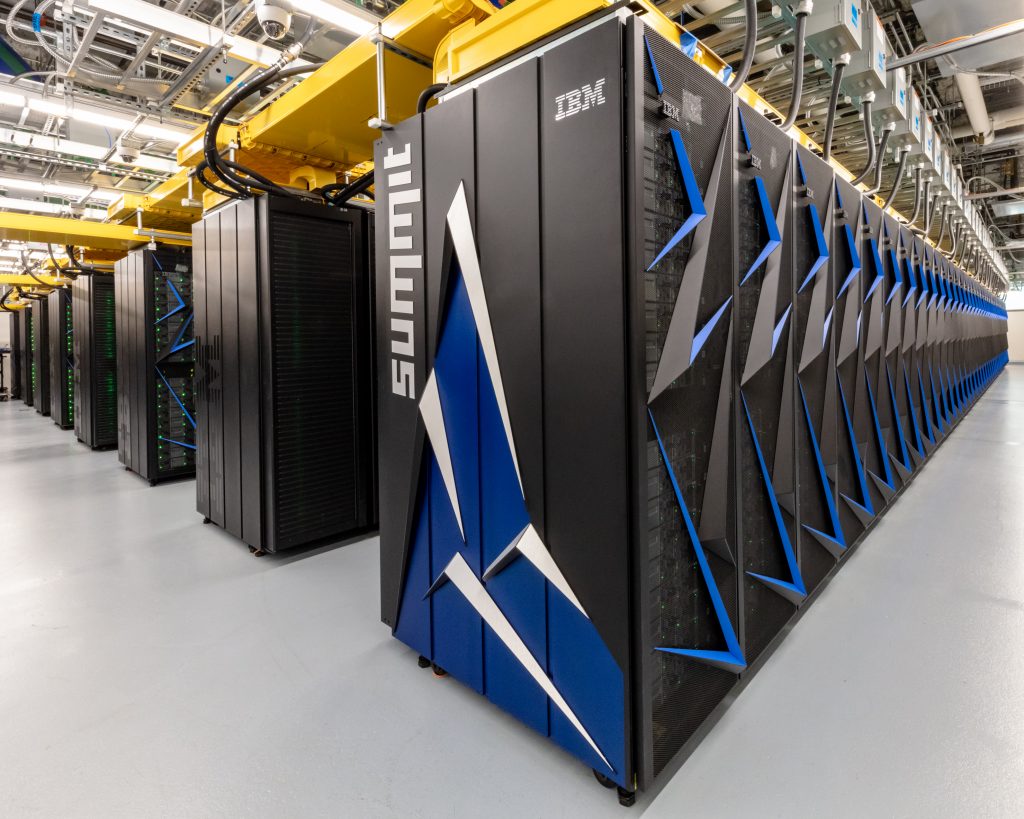
ORNL’s Summit supercomputer.
The team turned to ORNL for help from the Oak Ridge Leadership Computing Facility’s Summit supercomputer and from Ramki Kannan, an ORNL computational scientist and internationally known machine-learning expert.
Machine learning uses data to train an algorithm over time so the model improves with repetition and learns to recognize which solutions work best under varying conditions. Kannan and the GE team developed one of the first machine learning models that successfully tackles the near wall problem and the large eddy simulations needed to explore those complex physics.
The new model accounts for variables that can’t be easily reproduced in a lab, such as how fluids may flow around various shapes of turbine blades or how shock waves cause sudden changes in pressure and defy standard models.
“We wanted to go beyond existing approaches, and we knew machine learning could help us get there,” Priebe said. “We needed not just the computing power of Summit but the unique expertise that Ramki and his team could bring to this problem.”
Findings were published at the 2021 Institute of Electrical and Electronics Engineers International Conference on Big Data.
The computational power of Summit, then the fastest supercomputer in the U.S. at 200 petaflops — that’s 200 quadrillion calculations per second — enabled the team to run eight simulations of realistic conditions in a jet engine. The simulations relied on GE’s GENESIS code, developed and refined over the years by using Summit and its predecessors.
Those simulations generated about 1.1 terabytes of data — the equivalent of around 6.5 million pages of text or 500 hours of high-definition video — on turbulent flows. The team then ran Kannan’s machine learning model on Summit and used the simulation data to teach the model how to recognize turbulence patterns.
The level of detail used in the simulations created a rich pool of data that trained the model to make precise predictions about turbulence via deep learning, a form of machine learning that uses artificial neural networks similar to the human brain in their ability to draw conclusions from experience and to spot patterns.
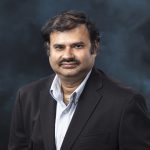
Ramki Kannan, ORNL computational scientist.
“It’s like how exercise produces muscle memory,” Kannan explained. “We’re training the model to spot these patterns on sight, the way you or I can immediately recognize a tree by its shape or tell a dog from a cat. We learn these patterns so well we don’t have to think about what we see because we know it instantly. We wanted the model to develop that same kind of memory and recognition for turbulence and its patterns.”
The study required 230,000 total node hours on Summit to simulate the various eddies and other possible flow patterns and then train the machine learning model.
“This was the first time we used machine learning to create a model of this type, which is a numerical technique for generating high-order solutions,” Kannan said. “Now the model knows these patterns so well it spots the turbulence pattern and gives you the most likely result without having to take these computationally intensive steps that would have been needed before. The model is widely applicable and can capture complex boundary layer physics under many different circumstances, so it can be used to study turbulence across a variety of technologies and conditions — from aerodynamics to power generation. We could not have reproduced these kinds of detailed patterns without a machine as fast and powerful as Summit.”
Tests found the model successfully captured complex processes such as the transition from laminar to turbulent flow, including every step in between — even the cascading eddies produced by the near wall problem.
“Summit has been a key technology lever for us, enabling us for the first time to capture these structures down to the surface level,” Priebe said.
The training on Summit prepared the model to run efficiently on smaller supercomputers, small servers and eventually even laptops — although at nowhere near the level of detail offered by Summit — for use by engineers across industries.
Priebe estimates the solution could lead to savings of $400 million annually. That’s equivalent to about 125 million gallons of jet fuel saved for U.S. airlines each year, for a reduction of roughly 850,000 metric tons in annual greenhouse gas emissions.
“The work we’re doing and hope to continue in exploring sustainable aviation fuels, hydrogen technologies and electric flights depends heavily on leveraging high-performance computing to push the boundaries of what’s doable, in and out of the lab,” GE spokesman Todd Alhart said. “Our partnership with ORNL has been important in helping us advance that body of work as we strive for higher performance and sustainability in our engines.”
The researchers plan to continue updating the model to set a new standard for accurate, cost-effective flow simulations.
“We haven’t put the model to work on an actual design project yet,” Kannan said. “Eventually, we hope to integrate this model inside a larger simulation. For example, we could replace sections of a GENESIS simulation with the model we developed to create a less computationally intensive alternative to current methods.”
Support for this research came from the DOE Office of Advanced Scientific Computing Research’s Leadership Computing Challenge and the Argonne Leadership Computing Facility’s Data Science Program. The OLCF and ALCF are Office of Science user facilities.
ORNL science writer Elizabeth Rosenthal contributed to this story.
Related publication: Rathakrishnan Baskaran, Ramakrishnan Kannan, Brian Barr, and Stephan Priebe, “Science-Guided Machine Learning for Wall-Modeled Large Eddy Simulation,” 2021 IEEE International Conference on Big Data (2021): 1809–1816, https://doi.org/10.1109/BigData52589.2021.9671436.
UT-Battelle manages ORNL for DOE’s Office of Science, the single largest supporter of basic research in the physical sciences in the United States. DOE’s Office of Science is working to address some of the most pressing challenges of our time. For more information, visit https://energy.gov/science.