Among the mysteries of science that continue to elude researchers, one stands apart in its vexing simplicity: ice. Yes, frozen water. Water’s transformation from liquid to solid is actually a complex process of nature that scientists have named—nucleation—yet do not fully understand. However, Princeton University researchers have taken trailblazing steps to study this phenomenon from the fundamental laws of nature using the Summit supercomputer at the Department of Energy’s (DOE’s) Oak Ridge National Laboratory (ORNL).
Through a combination of quantum mechanics and AI, the team has simulated how water molecules transition into solid ice at a level of accuracy and at a system size unmatched by previous methods. Their study, published in the journal Proceedings of the National Academy of Sciences, reveals the nucleation rates (how fast ice clusters form) in systems composed of hundreds of thousands of atoms. Such data could serve in a variety of initiatives, from improving the accuracy of weather and climate modeling to advancing the process of flash-freezing food.
So far, the Princeton team’s nucleation study is perhaps one of the most significant applications of DeePMD (named for deep potential molecular dynamics), a 2020 Gordon Bell Prize–winning simulation code optimized for high-performance computing (HPC). DeePMD uses quantum mechanics—the physical laws that determine how matter and energy interact on an atomic level—to describe the motion of atoms and molecules. Such first-principles simulations can produce more accurate results with greater predictive power than simpler, semi-empirical approaches, but at the cost of requiring much greater computational power.
“To study ice nucleation, we had to simulate large systems—a significant number of water molecules. It was impossible to do this in the past because there was no code able to perform such a big simulation with quantum accuracy,” said Pablo Piaggi, lead author and a postdoctoral research associate in Princeton’s chemistry department. “Having an HPC-optimized code and the Summit supercomputer gave us the opportunity to do groundbreaking science. We could have never done these simulations without them.”
Although Summit’s GPU-accelerated computing power helped speed up DeePMD’s large-scale, highly complex calculations, the code also employed deep neural networks, which are machine-learning algorithms most often used to recognize patterns in datasets of text, images, or sounds. The team trained a neural network in small molecular systems to identify atomic configurations with their corresponding forces. The neural network was then applied to larger systems to predict how their atoms will interact.
“Direct first-principles simulations are expensive to perform. This is because it’s hard to solve the equations that electrons obey, even if we resort to approximations. But with DeePMD, machine learning has come to the rescue with the ability to learn first-principles forces and to make them much cheaper to calculate,” said Piaggi, who performed the simulations on Summit and analyzed the results. “As a result, now we can tackle larger systems and for much longer times.”
Using quantum mechanics to predict the physical forces felt by atoms and molecules in molecular dynamics simulations was originally posited in 1985 by physicists Roberto Car and Michele Parrinello, who named their approach ab initio (Latin for “from the beginning”). Now, with the increase in computing power and the advent of AI, the promise of ab initio molecular dynamics is being fulfilled.
“In a sense, this is like a dream come true,” said Car, Princeton’s Ralph W. Dornte *31 Professor in Chemistry, who co-authored the study. “Our hope then was that eventually we could study systems like this one, but it was not possible without further conceptual development, and that development came via a completely different field—that of AI and data science.”
DeePMD’s development began in 2018 with a project led by Princeton graduate student Linfeng Zhang that applied deep neural networks to modeling quantum-mechanical interatomic forces. For its Gordon Bell Prize submission in 2020, DeePMD was tested on Summit in a project coordinated between Lawrence Berkeley National Laboratory; the University of California, Berkeley; Peking University; and Princeton.
For Princeton’s water-to-ice project, the team ran simulations on Summit of up to 300,000 atoms and used significantly less computing power than previously required and for much longer timespans.
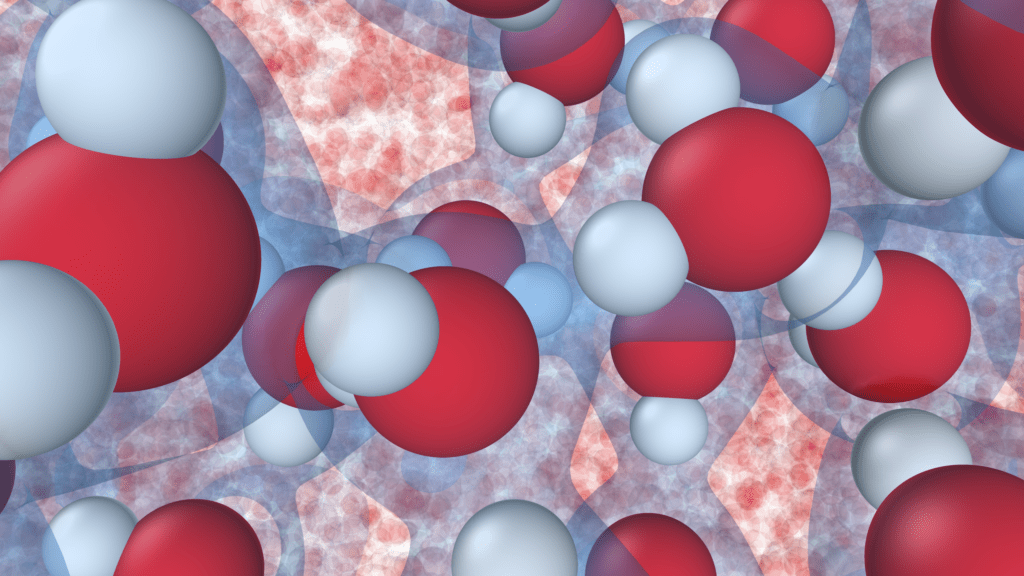
Researchers at Princeton University combined artificial intelligence and quantum mechanics to simulate what happens at the molecular level when water freezes. The result is the most complete yet simulation of the first steps in ice “nucleation,” a process important for climate and weather modeling. Image credit: Pablo Piaggi, Princeton University.
“This work provides one of the best studies yet of ice nucleation,” said Pablo Debenedetti, Princeton’s dean for research and a coauthor. “Ice nucleation is one of the major unknown quantities in weather prediction models. This is a quite significant step forward because we see very good agreement with experiments. We’ve simulated large systems, which were previously unthinkable for quantum calculations.”
Beyond the nucleation data the team reports, Piaggi thinks the project will stand as a significant advancement in adopting ab initio approaches in computational science.
“Our work is one of the first to simulate large systems with quantum accuracy to tackle an important problem. I believe it opens the path to many other exciting applications,” Piaggi said.
Related Publication
Pablo M. Piaggi, Jack Weis, Athanassios Z. Panagiotopoulos, Pablo G. Debenedetti, and Roberto Car, “Homogeneous Ice Nucleation in an Ab Initio Machine-Learning Model of Water,” Proceedings of the National Academy of Sciences 119, no. 33 (August 2022), /10.1073/pnas.2207294119.
UT-Battelle LLC manages ORNL for DOE’s Office of Science, the single largest supporter of basic research in the physical sciences in the United States. DOE’s Office of Science is working to address some of the most pressing challenges of our time. For more information, visit https://energy.gov/science.