A multidisciplinary team of researchers from the US Department of Energy’s (DOE’s) Oak Ridge National Laboratory (ORNL) and Purdue University won the Truth CT Reconstruction Grand Challenge, which was organized by the American Association of Physicists in Medicine (AAPM). The team’s win was announced at the 2022 AAPM Annual Meeting in Washington, DC, in July.
Using simulated, raw computed tomography (CT) scanner data supplied by the Duke University Center for Virtual Imaging Trials, 55 teams from around the world competed to reconstruct the highest resolution medical images with the most accurate clinical diagnosis for various pathological diseases. The challenge has direct applications to improving the identification of diseases and lowering doses of radiation during CT examinations.
“Better solutions for CT image reconstruction means higher-quality medical care,” said team leader Xiao Wang, a research scientist in the Multimodal Data Analytics Group at the Computational Sciences & Engineering Division (CSED) at ORNL. “Some lesions, such as early stage cancer, are hard to find and are often unnoticeable in clinical CT images. With better image reconstruction and clearer medical images, radiologists can more clearly identify the cancer nodules while minimizing radiation doses and radiation-induced cancer risks.”
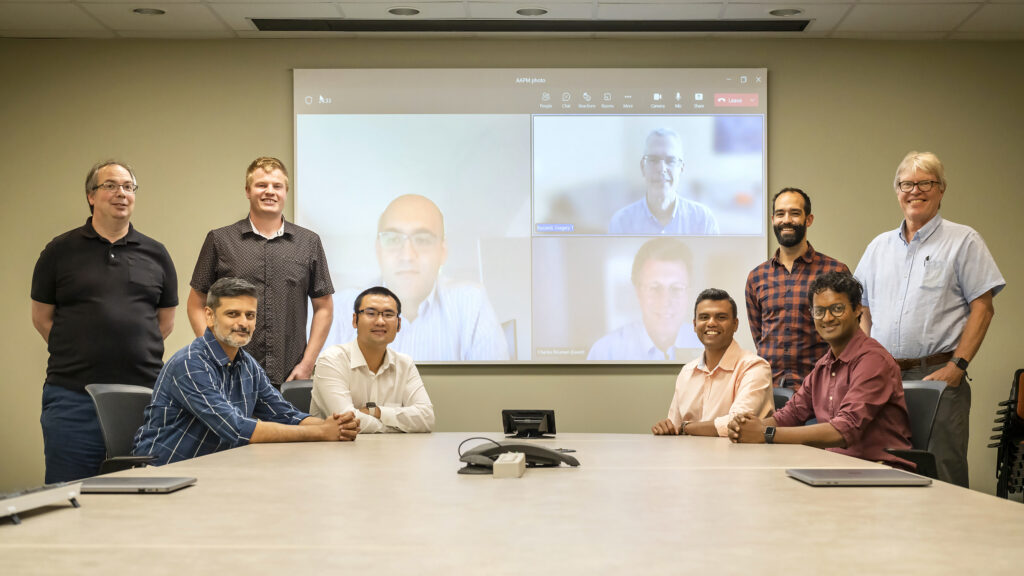
The winning team of the AAPM’s Truth CT Reconstruction Grand Challenge, from left: Balint Joo, Anuj Kapdia (leftmost sitting), Isaac Lyngaas, Xiao Wang, Amir Ziabari (on the screen, left, Gregery Buzzard (on the screen, top right), Charles Bouman (on the screen, bottom right), Murali Gopalakrishnan Meena, Matthew Norman, Singanallur Venkatakrishnan, Tom Beck. Photo by Carlos Jones/ORNL.
CT imaging uses x-rays, thus exposing patients to radiation. Higher x-ray exposure can produce clearer CT images—and a higher risk of radiation-induced cancer for the patient. Lower radiation exposure will reduce that risk, but it can also lead to poorer image quality. The AAPM’s CT Reconstruction Grand Challenge sought to find solutions to that conundrum: how can radiologists reconstruct images from raw scanner data acquired with low radiation doses while maintaining high image quality?
The ORNL/Purdue team won with a Bayesian estimation image reconstruction method called MBIR, or Model Based Iterative Reconstruction, which uses statistical and physics modeling to quantify uncertainties in the medical image acquisition and thus create a more advanced reconstruction. At a high level, MBIR jointly estimates three different models simultaneously: a physics-based data acquisition model that represents the precise scanner geometry, scanner movement, and x-ray attenuation information; a statistical noise model that describes the unknown errors in the raw scanner detector measurements and how these errors depend on the radiation dose; and an image prior model that promotes correct image appearance and texture while suppressing image artifacts.
Wang’s team developed this framework based on the MBIR method, which was originally created in the 1990s by Charles Bouman, the Showalter Professor of Electrical and Computer Engineering and Biomedical Engineering at Purdue University and Wang’s PhD advisor. The original MBIR is a general framework for reconstructing many kinds of tomographic images, with applications ranging from neuron imaging, security imaging, and industrial imaging to x-ray and electron microscopy.
However, medical CT scanning technology has evolved rapidly in the past 20 years with much more advanced acquisition physics and geometry, which made the original MBIR framework no longer applicable for state-of-the-art medical CT imaging systems. MBIR is also very computationally expensive and takes several days for a single image reconstruction.
Wang began working to rectify those shortcomings as a PhD student by developing a high-performance computing (HPC) solution for MBIR that leads to near-instantaneous image reconstruction and addresses the computation-time barrier for MBIR. This work was selected as a finalist for the 2017 ACM Gordon Bell Prize. During his postdoc years at Harvard Medical School, Wang redesigned the physics acquisition model for MBIR so it can reconstruct images from the newest medical CT scanner system and take advantage of its unique imaging acquisition physics.
Wang’s team continued this MBIR development for its winning AAPM Grand Challenge entry, which was a culmination of efforts from across ORNL divisions with researchers from CSED, the National Center for Computational Sciences (NCCS), and the Oak Ridge Leadership Computing Facility (OLCF) along with Purdue University collaborators and external imaging experts. The OLCF is a DOE Office of Science User Facility at ORNL.
“The collaborative interactions stemmed from a growing research effort that links artificial intelligence/machine learning methods and scalable HPC algorithms with cutting-edge imaging science applications,” said Thomas Beck, section head of Science Engagement at NCCS. “Dr. Wang did a great job leading the team to the successful outcome in a highly competitive challenge. The work will lead to the development of higher-resolution precision medicine driven by leadership-class computing facilities.”
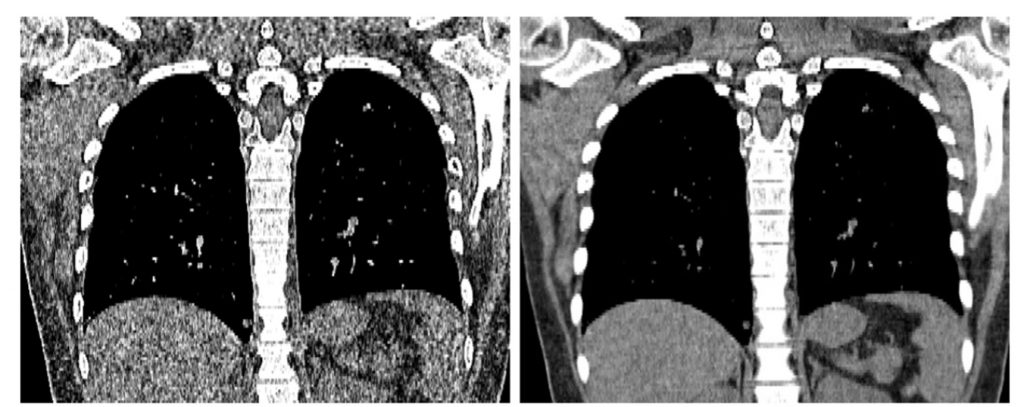
At left is a medical CT coronal image reconstructed by the state-of-the-art clinical image reconstruction software, Siemens ADMIRE. At right, the same image has been reconstructed by the Model-Based Iterative Reconstruction (MBIR) method develop by researchers at ORNL and Purdue University. The MBIR image has better image quality, clearer lesion detection, and less image noise. Image courtesy Xiao Wang/ORNL.
MBIR has been tested on patients using a Siemens SOMATOM Force CT scanner at Boston Children’s Hospital, and it produced superior image quality compared to the Siemens scanner alone. Wang and his team are conducting further testing and are developing advanced features for even more accurate medical imaging. A start-up company, High Performance Imaging LLC, is working with the team to commercialize MBIR for medical imaging applications.
Wang’s team members include ORNL researchers Tom Beck, Balint Joo, Anuj Kapadia, Isaac Lyngaas, Murali Gopalakrishnan Meena, Matthew Norman, Singanallur Venkatakrishnan, and Amir Ziabari, with Charles Bouman and Gregery Buzzard from Purdue University.
UT-Battelle LLC manages Oak Ridge National Laboratory for DOE’s Office of Science, the single largest supporter of basic research in the physical sciences in the United States. DOE’s Office of Science is working to address some of the most pressing challenges of our time. For more information, visit https://energy.gov/science.