The first step for biologists who want to develop new, more efficient biofuels is to understand the genetic underpinnings of plants that can be digested by microbes into chemical compounds. One species in particular, the black cottonwood tree, or Populus trichocarpa (poplar tree), has long been a focus of scientists interested in bioenergy.
To maximize poplar’s potential as a biofuel, researchers map the interactions between genes and expressed traits, or phenotypes, in order to predict how desired traits can be expressed based on the subject’s genetic makeup. With this knowledge, researchers can breed and genetically modify crops to maximize their potential as a biofuel.
However, this relationship between genes and their associated phenotypes often becomes complicated by phenomena like pleiotropy, which occurs when one gene is associated with two or more seemingly unrelated traits.
To unravel these complex interactions, Deborah Weighill and Daniel Jacobson enlisted the help of the Titan supercomputer at the Oak Ridge Leadership Computing Facility (OLCF), a US Department of Energy (DOE) Office of Science User Facility at DOE’s Oak Ridge National Laboratory (ORNL).
Working with the ORNL’s Center for Bioenergy Innovation (CBI), Weighill and her team used the now-decommissioned Cray XK7 supercomputer as well as the OLCF’s Eos cluster to analyze the results of a genome-wide association study (GWAS) which used genomic variants from the sequenced genomes of around 1,000 poplar trees to look for pleiotropic signatures—signatures of genes involved in multiple functions or associated with multiple different phenotypes.
In the study, the team developed and used a new process called multi-phenotype association (MPA) decomposition, a network-based method for characterizing the complex relationship between genetic variants and their associated phenotypes.
The study looked at the way single nucleotide polymorphisms (SNPs, pronounced “snips”), which are substitutions of a single base pair in DNA, are correlated with phenotypes and then clustered them based on these associated phenotypes. Specifically, the study mapped the relationship between SNPs and hundreds of individual metabolite levels.
“So, SNPs represent variants across our population of poplar trees,” Weighill said. “We cluster the SNPs so that what we get are groups of mutations that are grouped together if they’re associating with the same phenotypes in our poplar population.”
This clustering process involved taking a massive matrix of SNP-to-phenotype associations from the GWAS results and calculating the similarities between all pairs of SNPs.
Entering Powerset Space
Associating a gene with its multiple phenotypes helps to identify pleiotropy, but it doesn’t show the full genetic picture.
“In a gene-to-phenotype network,” Weighill said, “all we can see about genes is that they are connected to multiple phenotypes each. So, we can see they are pleiotropic in nature because they affect multiple phenotypes based on our GWAS results, but we can’t tell anything about which variants within that gene are affecting the phenotypes or what type of signature it is.”
To understand the topology of different phenotype associations within each gene, the MPA decomposition process forms what is called an association module, a discrete pairing of a single SNP and any phenotypes associated with that SNP.
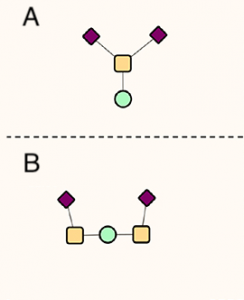
In A, the module (yellow) shows that both phenotypes (purple) interact with the same SNP. A researcher would be unable to modify the gene without affecting both phenotypes. In B, the presence of two separate modules indicates that modifying one SNP will only affect the associated phenotype.
“Modules allow us to a construct a new space, what we call powerset space.” Weighill said, “When we map genes into this space, it unravels the type of signature that the SNPs within that gene have. So that’s what MPA association is trying to do. It computationally and mathematically characterizes the different types of SNP-to-phenotype patterns so we can cluster genes not only based on what phenotypes they’re associating with but on the SNP-to-phenotype association pattern.”
Visualizing MPA signatures in the powerset space is important for researchers attempting gene modification experiments because they can gain an understanding of which genes could be potentially altered without affecting other phenotypes. As an added benefit, through MPA decomposition, biologists have a method for mining massive datasets to create numerically interpretable information from which they can derive hypotheses.
“Our aim is to help generate specific hypotheses that will move the field of bioenergy forward,” Weighill said. “We want to take data and then analyze it and encourage it into a format so that we can work together with biologists to formulate specific hypotheses on how to improve the poplar tree to be a more favorable bioenergy resource or identify new genes that are involved in functions that are of interest to the bioenergy research at CBI.”
The method developed by Weighill is not species specific, meaning that it can be applied to any organism as long as the mutations and phenotypes across its population have been measured. In the future, MPA decomposition can be used by researchers to extract meaningful information with enormous implications for biofuel development.
Also participating in the study were individuals from the University of Tennessee’s (UTK’s) Bredesen Center, ORNL’s Biosciences Division, UTK’s Department of Plant Sciences, West Virginia University’s Department of Biology, the DOE Joint Genome Institute, and HudsonAlpha Institute for Biotechnology.
Computing time was awarded through the OLCF Director’s Discretion program as well as the DOE INCITE program. Support for the Poplar GWAS dataset was provided by ORNL’s BioEnergy Science Center and the CBI, which are funded by the DOE Office of Science. The research also used resources from the ORNL Compute and Data Environment for Science (CADES) facility.
Related Publication: D. Weighill, P. Jones, C. Bleker, P. Ranjan, M. Shah, N. Zhao, M. Martin, S. DiFazio, D. Macaya-Sanz, J. Schmutz, A. Sreedasyam, T. Tschaplinski, G. Tuskan, and D. Jacobson, “Multi-Phenotype Association Decomposition: Unraveling Complex Gene-Phenotype Relationships.” Frontiers in Genetics 10 (2019): 417, doi:10.3389/fgene.2019.00417.
UT-Battelle LLC manages ORNL for the Department of Energy’s Office of Science, the single largest supporter of basic research in the physical sciences in the United States. The Office of Science is working to address some of the most pressing challenges of our time. For more information, please visit https://energy.gov/science.