Galen Shipman summarizes problems and solutions in HPCwire interview
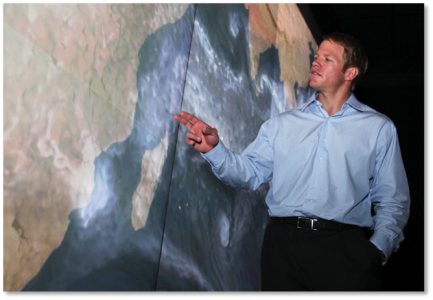
Galen Shipman, ORNL’s strategist for managing big data, points out a simulated storm threatening Madagascar. Models and simulations running on ORNL supercomputers reveal regional effects missed by less capable machines. The big climate data housed at the lab will soon exceed a petabyte.
Supercomputers at the Oak Ridge National Laboratory (ORNL) computing complex produce some of the world’s largest scientific data sets. Many are from studies using high-resolution models to evaluate climate change consequences and mitigation strategies. The Department of Energy (DOE) Office of Science’s Jaguar (the pride of the Oak Ridge Leadership Computing Facility, or OLCF), the National Science Foundation (NSF)-University of Tennessee’s Kraken (NSF’s first petascale supercomputer), and the National Oceanic and Atmospheric Administration’s Gaea (dedicated solely for climate modeling) all run climate simulations at ORNL to meet the science missions of their respective agencies. Such simulations reveal Earth’s climate past, for example as described in a 2012 Nature article that was the first to show the role carbon dioxide played in helping end the last ice age. They also hint at our climate’s future, as evidenced by the major computational support that ORNL and Lawrence Berkeley National Laboratory continue to provide to U.S. global modeling groups participating in the upcoming Fifth Assessment Report of the United Nations Intergovernmental Panel on Climate Change. Remote sensing platforms such as DOE’s Atmospheric Radiation Measurement facilities, which support global climate research with a program studying cloud formation processes and their influence on heat transfer, and other climate observation facilities, such as DOE’s Carbon Dioxide Information Analysis Center at ORNL and the ORNL Distributed Active Archive Center, which archives data from the National Aeronautics and Space Administration’s Earth science missions, generate a wide variety of climate observations. Researchers at the Oak Ridge Climate Change Science Institute (ORCCSI) use coupled Earth system models and observations to explore connections among atmosphere, oceans, land, and ice and to better understand the Earth system. These simulations and climate observations produce big data that must be transported, analyzed, visualized, and stored. HPCwire sat down with Galen Shipman, data systems architect for ORNL’s Computing and Computational Sciences Directorate, who oversees data management at the OLCF, to discuss strategies for coping with the “3 Vs”—variety, velocity, and volume—of the big data that climate science generates.
HPCwire: Why do climate simulations generate so much data?
Shipman: The I/O [input/output] workloads in many climate simulations are based on saving the state of the simulation, the Earth system, for post analysis. Essentially, they’re writing out time series information at predefined intervals—everything from temperature to pressure to carbon concentration, basically an entire set of concurrent variables that represent the state of the Earth system within a particular spatial region. If you think of, say, the atmosphere, it can be gridded around the globe as well as vertically, and for each subgrid, we’re saving information about the particular state of that spatial area of the simulation. In terms of data output, this generally means large numbers of processors concurrently writing out system state from a simulation platform such as Jaguar. Many climate simulations output to a large number of individual files over the entire simulation run. For a single run you can have many files created which when taken in aggregate can exceed several terabytes. Over the past few years, we have seen these dataset sizes increase dramatically. Climate scientists, led by ORNL’s Jim Hack, who heads ORCCSI and directs the National Center for Computational Sciences, have made significant progress in increasing the resolution of climate models, both spatially and temporally along with increases in physical and biogeochemical complexity, resulting in significant increases in the amount of data generated by the climate model. Efforts such as increasing the frequency of sampling in simulated time are aimed at better understanding aspects of the climate such as the daily cycle of the Earth’s climate. Increased spatial resolution is of particular importance when you’re looking at localized impacts of climate change. If we’re trying to understand the impact of climate change on extreme weather phenomena, we might be interested in monitoring low-pressure areas, which can be done at a fairly coarse spatial resolution. But if you want to identify a smaller-scale low-pressure anomaly like a hurricane, we need to go to even higher resolution, which means even more data is generated with more analysis required of that data following the simulation. In addition to higher-resolution climate simulations, a drive to better understand the uncertainty of a simulation result, what can naively be thought of as putting “error bars” around a simulation result, is causing a dramatic uptick in the volume and velocity of data generation. Climate scientist Peter Thornton is leading efforts at ORNL to better quantify uncertainty in climate models as part of the DOE Office of Biological and Environmental Research (BER) funded “Climate Science for a Sustainable Energy Future” project. In many of his team’s studies, a climate simulation may be run hundreds, or even thousands of times, each with slightly different model configurations in an attempt to understand the sensitivity of the climate model to configuration changes. This large number of runs is required even when statistical methods are used to reduce the total parameter space explored. Once simulation results are created, the daunting challenge of effectively analyzing them must be addressed.
HPCwire: What is daunting about analysis of climate data?
Shipman: The sheer volume and variety of data that must be analyzed and understood is the biggest challenge. Today, it is not uncommon for climate scientists to analyze multiple terabytes of data, spanning thousands of files, across a number of different climate models and model configurations, in order to generate a scientific result. Another challenge that climate scientists are now facing is the need to analyze an increasing variety of datasets, not simply simulation results, but also climate observations often collected from fixed and mobile monitoring. The fusion of climate simulation and observation data is being driven to develop increasingly accurate climate models and to validate this accuracy using historical measurements of the Earth’s climate. Conducting this analysis is a tremendous challenge, often requiring weeks or even months using traditional analysis tools. Many of the traditional analysis tools used by climate scientists were designed and developed over two decades ago when the volume and variety of data that scientists must now contend with simply did not exist. To address this challenge, DOE BER began funding a number of projects to develop advanced tools and techniques for climate data analysis, such as the Ultrascale Visualization Climate Data Analysis Tools (UV-CDAT) project, a collaboration including Oak Ridge National Laboratory, Lawrence Livermore National Laboratory, the University of Utah, Los Alamos National Laboratory, New York University, and KitWare, a company that develops a variety of visualization and analytic software. Through this project we have developed a number of parallel analysis and visualization tools specifically to address these challenges. Similarly, we’re looking at ways of integrating this visualization and analysis toolkit within the Earth System Grid Federation, or ESGF, a federated system for managing geographically distributed climate data, of which ORNL is a primary contributor. The tools developed as a result of this research and development are used to support the entire climate science community. While we have made good progress in addressing many of the challenges in data analysis, the geographically distributed nature of climate data, with archives of data spanning the globe, presents other challenges to this community of researchers.
To read the complete interview on the HPCwire website, please see: https://www.hpcwire.com/hpcwire/2012-08-15/climate_science_triggers_torrent_of_big_data_challenges.html