AI Surrogates for HPC
February 27, 2025 | 1:00–2:30 PM EST
Location: Zoom
Spring 2025 AI Focused Frontier Hackathon & Frontier AI Training Series
Register HereEvent Description: Join us for our second session on “AI Surrogates for HPC,” where we’ll explore the potential of artificial intelligence to aid HPC and drive science. This session will include expert-led discussions, practical examples, and time to share your own HPC + AI efforts with our staff and mentors.
Bonus Opportunity: This event qualifies for a Bonus Point on your Frontier AI Hackathon Participation Proposal form. See https://www.olcf.ornl.gov/calendar/spring-2025-ai-focused-frontier-hackathon/
Interactive Discussion: Bring your own HPC + AI challenges and ideas to discuss with our experienced staff and mentors. This is a unique opportunity to exchange knowledge, brainstorm solutions, and explore collaborations.
Who Should Attend:
- Researchers, developers, and students working on or interested in AI and HPC
- Participants considering participation in the April Frontier AI Hackathon
- Anyone curious about applying AI to challenging scientific problems
Topics Covered:
-
Source Term Estimation Problem:
- An inverse problem tackles the challenge of working backward from observed effects to deduce their causes. Imagine sensors tracking oil spread in the ocean: how do we determine where the spill originated? In this session, we’ll simplify this concept using a 2D heat conduction example: identifying the source of heat on a thin, flat plate based on temperature readings from sensors. Learn how AI can rapidly solve such problems with a single run, bypassing the time-consuming process of iterative simulations.
-
Interpretability of Surrogate Models:
- Neural networks can feel like black boxes, but interpretability techniques reveal how inputs influence outputs, providing transparency and trust in AI predictions. Discover tools and methods that make surrogate models understandable and actionable, essential for high-stakes applications.
-
Bayesian Neural Networks
- Bayesian Neural Networks offer a powerful framework for making predictions while understanding the associated uncertainties, making them indispensable in critical applications where decision-making confidence is paramount.
- As opposed to regular Neural Networks, where the weights of the network are scalar numbers, Bayesian Neural Networks (BNNs) represent the weights as probability distributions.
- By doing so, BNNs return a valid prediction, along with a measure of how uncertain that prediction is.
- By accounting for uncertainty, BNNs can prevent overfitting to limited data and provide more reliable and interpretable results, enhancing trust in AI systems.
- Bayesian Neural Networks offer a powerful framework for making predictions while understanding the associated uncertainties, making them indispensable in critical applications where decision-making confidence is paramount.
-
SmartSim:
- SmartSim will give an overview of their past recorded AI Surrogate trainings from the OLCF AI series. Those trainings cover the SmartSim software and many examples of how AI surrogates can enhance HPC.
SmartSim Training
Date | Training | Presenter | Presentation Materials |
---|---|---|---|
2024-09-26 | AI Training Series: SmartSim 2024 Applications in climate modeling and CDF Applications in Molecular Dynamics and CFD Building Simple Smart Sim Applications | Andrew Shao (HPE) | ( recording | slides | tutorial ) |
2023-07-13 | AI Training Series: SmartSim at OLCF | Andrew Shao, Matt Ellis, Matt Drozt (HPE) | ( recording | slides | tutorial | Q&A ) |
2022-03-30 | Machine Learning for HPC simulations: Using PyTorch, TensorFlow in Fortran, C, and C++ with SmartSim | Sam Partee (HPE) | ( presentation ) |
Training Materials
( Recording | Slides )
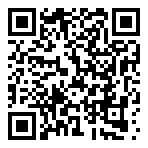