A research team led by the University of Maryland has been nominated for the Association for Computing Machinery’s Gordon Bell Prize. The team is being recognized for developing a scalable, distributed training framework called AxoNN, which leverages GPUs to rapidly train large language models, or LLMs.
Winners of the Gordon Bell Prize will be announced at the International Conference for High Performance Computing, Networking, Storage, and Analysis in Atlanta, Georgia, on Nov. 17 to 22.
The immense computational power of Oak Ridge National Laboratory’s Frontier supercomputer and its thousands of GPUs showcased the framework’s remarkable scalability and efficiency, both of which enable AxoNN to successfully benchmark AI model training with up to 320 billion parameters at record-breaking speeds. Frontier is managed by the Oak Ridge Leadership Computing Facility, a Department of Energy Office of Science user facility.
“Training or fine-tuning LLMs requires tremendous amounts of computing resources. Leveraging powerful GPU accelerators in exascale machines is the key to developing the models faster and more efficiently,” said Abhinav Bhatele, associate professor and director of the Parallel Software and Systems Group at the University of Maryland.
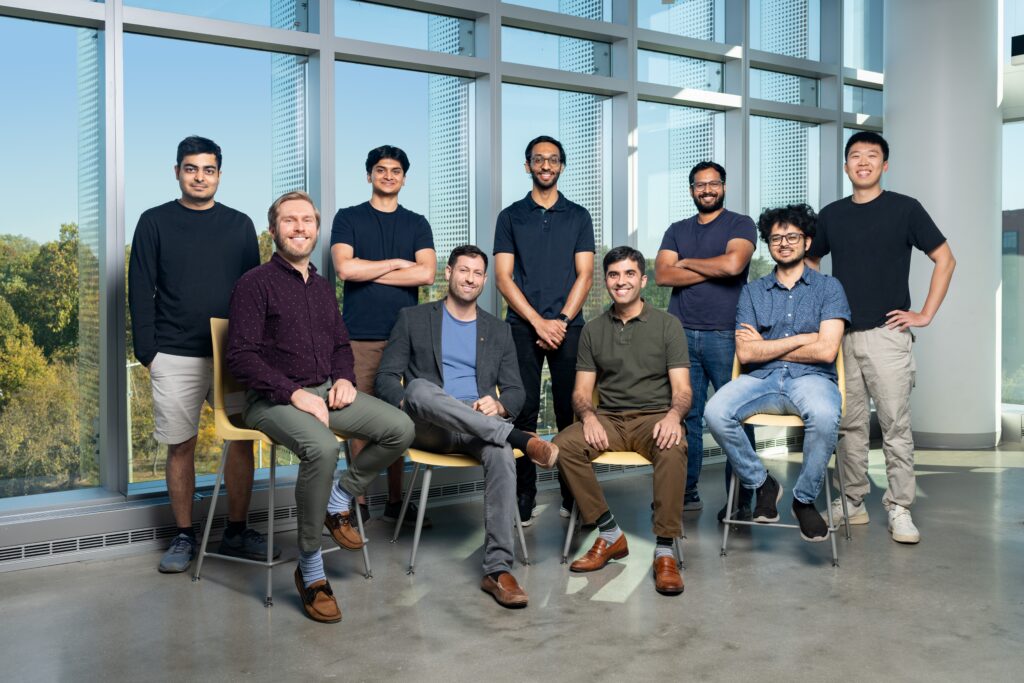
UMD team members nominated for the Gordon Bell Prize include (from left to right) Abhimanyu Hans, John Kirchenbauer, Aditya Ranjan, Tom Goldstein, Prajwal Singhania, Abhinav Bhatele, Neel Jain, Siddharth Singh and Yuxin Wen. Not pictured is Manli Shu. Credit: Mike Morgan/University of Maryland
LLMs are the computational models that drive chat bots, including platforms such as OpenAI’s ChatGPT and Microsoft’s CoPilot. These models are based on datasets with billions and trillions of “weights” — the values of which are used to determine how important a word or piece of information is and how best to answer questions.
AxoNN is a highly scalable open-source framework based on the PyTorch machine-learning library and is designed to parallelize the training and fine-tuning of LLM models across tens of thousands of GPUs. AxoNN is based on a hybrid parallel algorithm that uses a 3D matrix multiplication algorithm first described in the first described in the IBM Journal of Research and Development in 1995.
The computational power of the Frontier supercomputer — currently ranked No. 1 on the TOP500 list of the world’s fastest supercomputers — comes in part from the unique design of its more than 37,000 AMD Instinct™ MI250X GPUs.
Using approximately 16,000 of Frontier’s AMD GPUs, AxoNN clocked an unprecedented speed of 1.38 exaflops per second when running at a reduced precision. One exaflop is more than a quintillion, or a billion-billion, calculations per second.
Details about the team’s achievement can be found in their preprint publication, “Democratizing AI: Open-source Scalable LLM Training on GPU-based Supercomputers.”
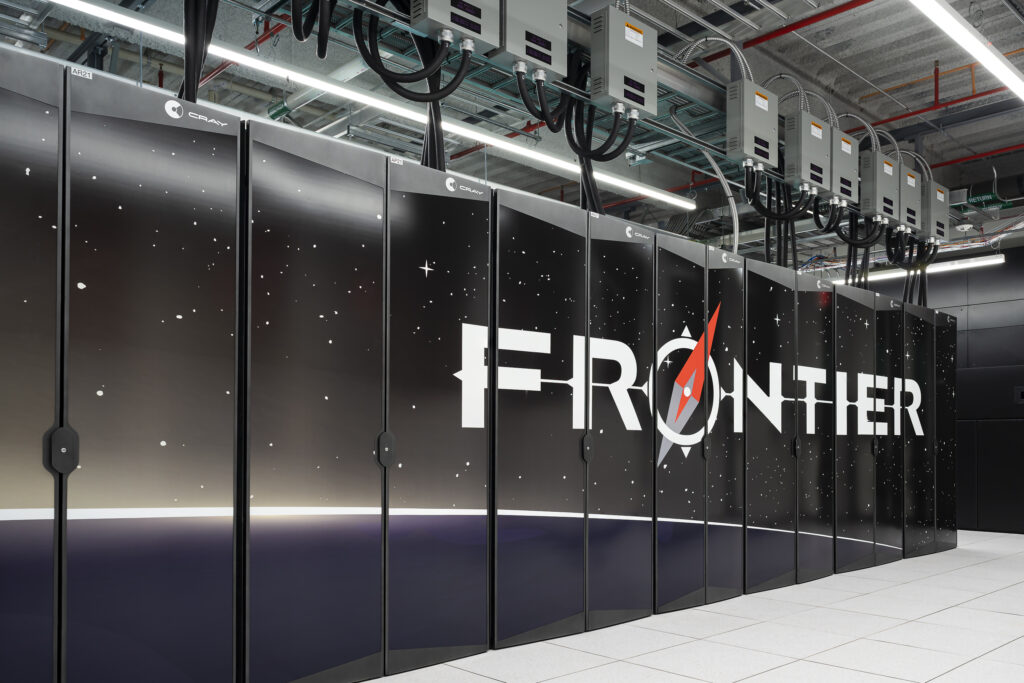
This year, four of the six teams nominated for Gordon Bell Prize awards used the Frontier supercomputer. Credit: Carlos Jones/ORNL, U.S. Dept. of Energy
Frontier’s computing power also allowed the team to study LLM memorization behavior at a larger scale than ever before. They found that privacy risks associated with problematic memorization tend to occur in models with greater than 70 billion parameters.
“Generally, the more training parameters there are, the better the LLM will be,” Bhatele said. “However, introducing more training parameters also increases privacy issues and copyright risks caused by memorization of training data that we don’t want the LLMs to regurgitate. My colleague and co-author, Tom Goldstein, has coined a term for it: catastrophic memorization.”
To mitigate the problem, they used a technique called Goldfish Loss that randomly omits certain bits of information during training and prevents the model from memorizing entire sequences that could contain sensitive or proprietary data. Frontier’s scalability allowed the team to test the mitigation strategy efficiently in large experiments with models of different sizes up to 405 billion parameters.
“We are extremely pleased with AxoNN’s performance on Frontier and the other leadership systems we used in our experiments,” Bhatele said. “Being recognized for this achievement is extra special, and everyone on the team is excited about our presentation at the upcoming supercomputing conference.”
The study also used the Alps supercomputer at the Swiss National Supercomputing Centre in Lugano, Switzerland, and the Perlmutter supercomputer at DOE’s National Energy Research Scientific Computing Center, an Office of Science user facility located at Lawrence Berkeley National Laboratory.
The Gordon Bell effort on AxoNN was led by Siddharth Singh, a senior doctoral student in Bhatele’s group, which also includes Prajwal Singhania and Aditya Ranjan. Other collaborators include Tom Goldstein, John Kirchenbauer, Yuxin Wen, Neel Jain, Abhimanyu Hans, and Manli Shu (University of Maryland); Jonas Geiping (Max Planck Institute for Intelligent Systems); and Aditya Tomar (UC Berkeley).
Access to high-performance computing resources at ORNL were awarded through DOE’s Innovative and Novel Computational Impact on Theory and Experiment program.
UT-Battelle manages ORNL for DOE’s Office of Science, the single largest supporter of basic research in the physical sciences in the United States. DOE’s Office of Science is working to address some of the most pressing challenges of our time. For more information, visit energy.gov/science.