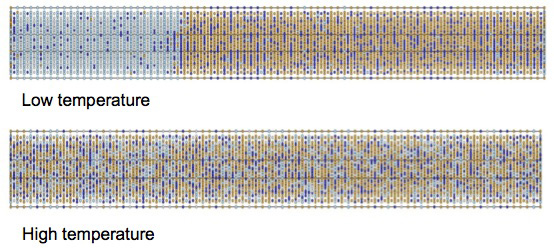
Results of large scale simulations showing the Alnico alloy separates into FeCo-rich and NiAl-rich phases at low temperatures and is a homogenized phase at high temperatures.
Simulations could uncover competitive substitutes for these super strong magnets
Rare earth metals—a group of elements identified primarily as lanthanides on the periodic table—are used to manufacture exceptionally strong permanent magnets.
These magnets (most often containing the rare earth element neodymium) are in high demand for machines such as motors and car engines, wind turbine generators, and magnetic resonance imaging machines—to name a few. Their stability and long-term performance are especially useful in machines operating at inaccessible locations, such as in off-shore wind turbines, and in devices too expensive to easily repair. Compared to the ferrite magnets that decorate refrigerator doors, neodymium magnets can be four times as magnetic and seven times less likely to become demagnetized.
However, environmental concerns over mining rare earth metals, their costs, and an unpredictable supply chain are making them less and less attractive to American manufacturers. Rare earth ores can contain traces of radioactive elements like uranium, and the ore must be processed using a mixture of toxic chemicals. Despite these environmental concerns, rising demand has driven up their price. Rare earths are more sporadically distributed across the Earth’s surface than iron ore and other metals, making them expensive to mine, and an unbalanced market (nearly 90 percent of rare earths are mined in China) further impacts availability. In fact, high demand in the United States led to the reopening of the Mountain Pass mine in California in 2012 after a decade-long hiatus.
For its part, the US Department of Energy (DOE) is also mining for solutions to the rare earth problem—but with high-performance computing instead of bulldozers. Researchers are using the hybrid CPU–GPU, 27-petaflop Titan supercomputer managed by the Oak Ridge Leadership Computing Facility (OLCF) at DOE’s Oak Ridge National Laboratory (ORNL) to discover alternative materials that can substitute for rare earths. The OLCF is a DOE Office of Science User Facility.
“There is a strong desire to find new magnetic materials that are equally as useful as neodymium-based magnets but are cheaper or less dependent on a single supplier,” said ORNL’s Markus Eisenbach.
Bruce Harmon of Ames National Laboratory’s Division of Materials Science and Engineering is a project leader using Titan to create computational methods for exploring material structure and composition in non–rare earth permanent magnets.
“We’re very much working in collaboration with experimentalists, but what we can’t do in experiment that we can simulate on Titan is the interaction among atoms,” Harmon said. “We can improve materials much faster if we know how atoms are interacting to generate strong magnetic moments.”
In addition to being influenced by a complex landscape of atomic interactions, magnetic materials are also influenced by the heat treatments they undergo during manufacture.
“When different constituents are mixed, melted, and cooled, then solidified and heat-treated at different times and temperatures, it is complicated to understand how the material is responding,” Eisenbach said.
While some HPC simulations, such as molecular dynamics simulations for drug design, can replicate the behavior of individual atoms in a cluster of molecules, materials research usually necessitates the study of larger chunks of material over longer timescales to simulate the effects of processing and manufacture on structure and stability.
With a 2013 INCITE award, the team used the computational method they developed to study the stability of Alnico at high temperatures. Alnico is a common class of alloys for non–rare earth permanent magnets comprised of aluminum, nickel, cobalt, and iron.
While experimentalists have been able to determine an underlying cubic structure of Alnico, it is more difficult to observe where its chemical elements are located within this structure. The Alnico elements nickel and cobalt are transition metals, as is iron, which is often added to improve the magnetic properties. These transition metal elements are not easily distinguished by x-ray diffraction experiments used to study 3D atomic arrangements.
“Theoretical work is crucial to understanding the best atomic arrangements so that we can optimize this system for better performance in permanent magnet applications,” Harmon said.
Harmon’s team used an adaptive genetic algorithm they developed to calculate the atomic and energy structure of Alnico in its ground state. By first calculating a material’s ground-state structure, or the structure it retains at its lowest possible energy, researchers can begin simulating changes to the material’s magnetic structure as its physical structure undergoes changes such as those imposed during processing.
They initially tested their model by calculating the ground-state structure of a thoroughly observed neodymium compound, Nd2Fe14B4, which has 68 atoms in its periodic unit cell. (The unit cell is the smallest sample of a repeating structure within a material.)
The adaptive genetic algorithm starts out with a population of possible arrangements, calculates the energy of each arrangement, and determines the “fittest” arrangements close to the lowest energy value. Imitating the process of natural selection, the algorithm continues this process through many iterations, or “generations,” until the likely ground-state structure is found.
Alnico itself has a complex, quasi-crystal structure and is often alloyed with iron to optimize its use in permanent magnets. The team simulated the Alnico alloy being fused through heat treatments by incorporating rising temperatures into their models
“We’re trying to see what’s happening at 600, 900, and 1,300 degrees Celsius,” Harmon said.
The group used a unit cell containing 3,380 sites to study these high-temperature phases. Because the computational load would be too expensive to model such a large unit cell at many incremental temperatures, the team is using a Monte Carlo method that relies on statistical probability to estimate how atoms in a large unit cell are arranged at different temperatures.
“Monte Carlo calculations can investigate how the material formation takes place and what its different configurations are,” said Eisenbach, who helped Harmon implement a new version of the Monte Carlo simulation to accelerate their research using Titan’s GPUs.
The team’s simulations matched experimental results that show an Alnico compound containing iron, cobalt, nickel, and aluminum separates into FeCo-rich and NiAl-rich phases at lower temperatures, whereas the alloy is stable at higher temperatures.
“We’re beginning to see the theoretical simulations supporting experimental results, which shows that our models are accurate and we can identify structural motifs that help understand the magnetic behavior of these rare earth substitutes,” Harmon said.
Related Publications:
- Xin Zhao, Qiang Shu, Manh Cuong Nguyen, Yangang Wang, Min Ji, Hongjun Xiang, Kai-Ming Ho, Xingao Gong, and Cai-Zhuang Wang, “Interface Structure Prediction from First-Principles,” J. Phys. Chem. C 118, 9524 (2014).
- Aaron Gilad Kusne, Tieren Gao, Apurva Mehta, Liqin Ke, Manh Cuong Nguyen, Kai-Ming Ho, Vladimir Antropov, Cai-Zhuang Wang, Matthew J. Kramer, Christian Long, and Ichiro Takeuchi, “On-the-fly machine learning for high-throughput experiments: search for rare-earth free permanent magnets,” Sci. Rep. 4, 6367 (2014).
Oak Ridge National Laboratory is supported by the US Department of Energy’s Office of Science. The single largest supporter of basic research in the physical sciences in the United States, the Office of Science is working to address some of the most pressing challenges of our time. For more information, please visit science.energy.gov