Speed, algorithm improvements make team finalist for Gordon Bell Prize
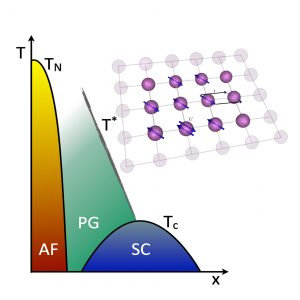
Generic temperature (T) vs. doping (x) phase diagram of the cuprates with antiferromagnetic (AF), superconducting (SC) and pseudogap (PG) phases. The inset on the top right shows an embedded Hubbard cluster representing a DCA calculation of a Hubbard model on a square lattice with nearest neighbor hopping t and local Coulomb repulsion U.
A team of researchers simulating high-temperature superconductors has topped 15 petaflops—or 15 thousand trillion calculations a second—on Oak Ridge National Laboratory’s (ORNL’s) Titan supercomputer. More importantly, they did it with an algorithm that substantially overcomes two major roadblocks to realistic superconductor modeling.
For their achievement, the team from ETH Zurich in Switzerland and ORNL was named a finalist for the Gordon Bell Prize, awarded each year for “outstanding achievement in high-performance computing.”
Materials become superconducting when electrons within them form pairs—called Cooper pairs—allowing them to collect into a condensate. As a result, superconducting materials conduct electricity without resistance, and therefore without loss. This makes them immensely promising in energy applications such as power transmission. They are also especially powerful magnets, a property exploited in technologies such as maglev trains and MRI scanners.
The problem with these materials is that they are superconducting only when they are very, very cold. For instance, the earliest discovered superconductor, mercury, had a transition temperature of 4.2 Kelvin, which is below -450 degrees Fahrenheit and very close to absolute zero. Mercury and other early superconductors were cooled with liquid helium—a very expensive process. Later materials remained superconducting above liquid nitrogen’s boiling point of −321 degrees Fahrenheit, making their use less expensive.
The discovery, or creation, of superconductors that needn’t be cooled would revolutionize power transmission and the energy economy.
The Swiss and American team approaches the problem with an application called DCA++, with DCA standing for “dynamical cluster approximation.” DCA++ simulates a cluster of atoms using the Hubbard model—which describes the behavior of electrons in a solid. It does so with a quantum Monte Carlo technique, which involves repeated random sampling.
The application earned its development team the Gordon Bell Prize in 2008.
The new method, known as DCA+, was developed largely by Peter Staar at ETH Zurich. It scaled to the full 18,688-node Titan system and took full advantage of the system’s NVIDIA GPUs, reaching 15.4 petaflops.
In addition, it takes full advantage of the energy efficiency inherent in Titan’s hybrid architecture. Each node of Titan contains both a CPU and a GPU. Using this system, simulation of the team’s largest realistic clusters consumed 4,300 kilowatt-hours. The same simulation on a comparable CPU-only system, the Cray XE6, would have consumed nearly eight times as much energy, or 33,580 kilowatt-hours.
The DCA+ algorithm also took a bite out of two nagging problems common to dynamic cluster quantum Monte Carlo simulations. These are known as the fermionic sign problem and the cluster shape dependency.
The sign problem is a major complication in the quantum physics of many-particle systems when they are modeled with the Monte Carlo method.
Particles in quantum mechanics are described by a wave function. For electrons and other fermions, this function switches from positive to negative—or vice versa—when two particles are interchanged. If you then sum the many-particle states, the positive and negative values nearly cancel one another out, essentially destroying the accuracy of the simulation.
“This is a cluster method,” said team member Thomas Maier of ORNL. “If you could make the cluster size infinite, then you would get the exact solution. So the goal is to make it as large as possible.
“But there’s a problem when you deal with electrons, which are fermions. It’s the infamous fermion sign problem, and it really limits the cluster size we can go to and the lowest temperature we can go to with quantum Monte Carlo.”
The sign problem cannot be overcome simply by creating larger supercomputers, Maier noted, because computational demands grow exponentially with the number of atoms being simulated. In other words, as you go to realistically large systems, you get problems that overwhelm not only every existing supercomputer, but any system we’re likely to see in the foreseeable future.
According to team member Thomas Schulthess of ETH Zurich and ORNL, the DCA+ algorithm arrives at a solution nearly 2 billion times faster than its DCA predecessor. So while it doesn’t make the sign problem go away entirely, it does make room for much more useful simulations, specifically by allowing for more atoms at lower temperatures—a key requirement, since so far superconductivity happens only in very cold environments.
The other problem—cluster shape dependency—meant that when the researchers simulated an atom cluster, the answer they got varied widely depending on the shape of the cluster.
“Let’s say you have two 16-site clusters,” Maier explained, “one two-dimensional system with a four-by-four cluster and another 16-site cluster of a different shape. The results with the standard DCA method depended a lot on the cluster shape. That’s of course something you don’t really want.
“By improving the algorithm we succeeded in getting rid of this cluster shape dependence. Before you would get vastly different results for the superconducting transition temperature, but now you get pretty much the same.”
The reduced sign problem, combined with the power of Titan, also allows the group to simulate much larger systems. In the past, the group was limited to eight-atom cluster simulations if it wanted to get down to the transition temperature for realistic parameters. More recently it has been able to scale up to 28-atom systems.
As the team moves forward, Maier noted, it would like to simulate more complex and realistic systems. For instance, two of the most promising materials in high-temperature superconducting research, which contain copper and iron, hold their electrons in a number of different orbitals. Yet, so far the team has simulated only one of these orbitals.
“One direction we want to go into is to make the models more realistic by including more degrees of freedom, or orbitals. But before you do that you want to have a method that allows you to get an accurate answer for the simple model. Then you can move on to more complicated models.”
“The question is always, ‘Do you get to the interesting region where you get interesting physics before you hit the sign problem,’” Maier noted. “We were able to get there to some extent before we had this new method. But now we really have a significant improvement. Now we can really look at realistic parameters.”
The Gordon Bell Prize will be presented November 21 during the SC13 supercomputing conference in Denver. Besides Staar, Maier, and Schulthess, the DCA+ team includes Raffaele Solca and Gilles Fourestey of ETH Zurich and Michael Summers of ORNL.